Origin E8
AI Inference for the Highest Performing Systems
From data centers to autonomous cars, the most demanding AI applications need high-performance NPUs with the lowest possible latency. With its highly customizable architecture, the Origin™ E8 delivers performance that scales to 128 TOPS in a single core and PetaOps with multiple cores.
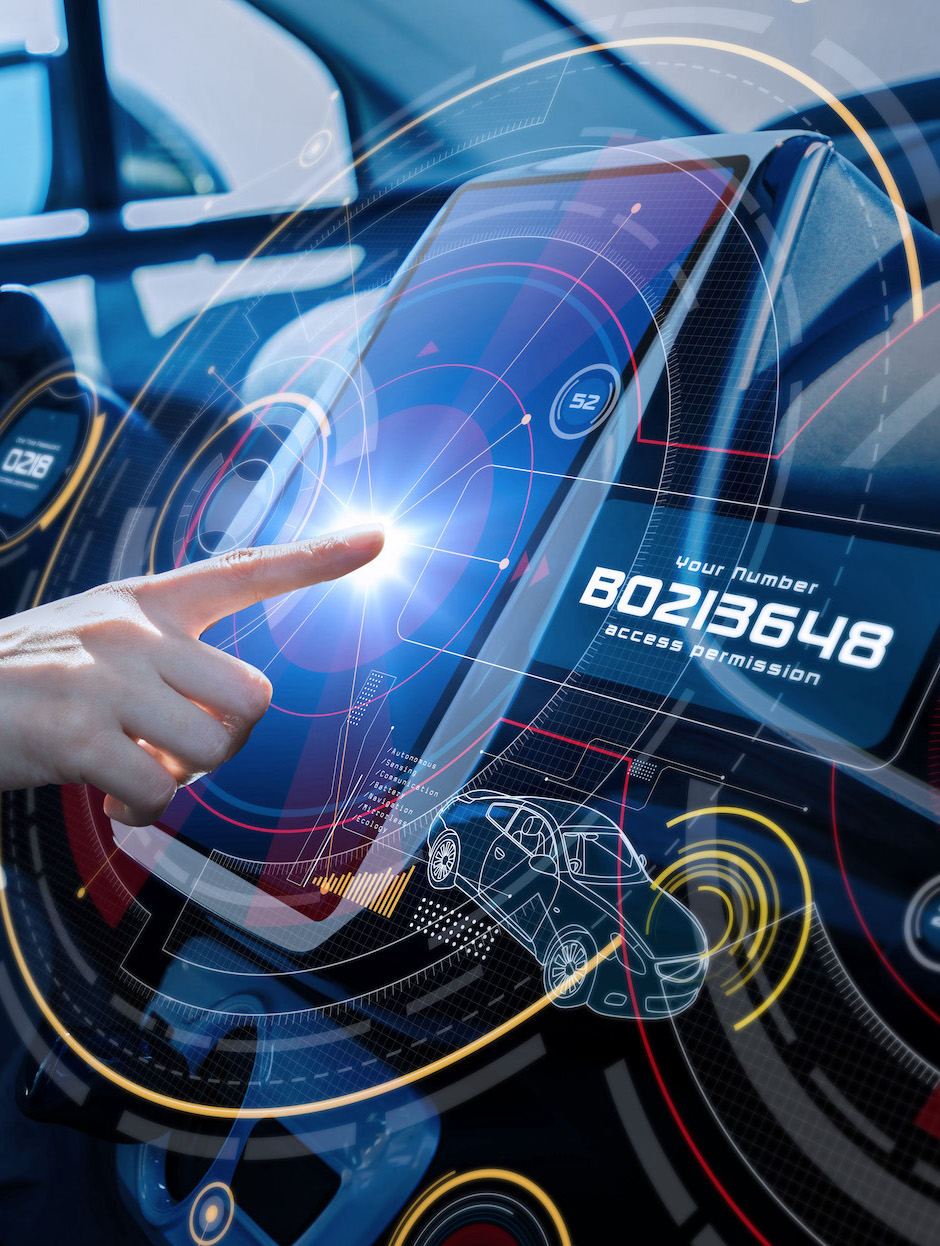
A Top Performer
The Origin E8 is a family of NPU IP inference cores designed for the most performance-intensive applications, including automotive and data centers. With its ability to run multiple networks concurrently with zero penalty context switching, the E8 excels when high performance, low latency, and efficient processor utilization are required. Unlike other IPs that rely on tiling to scale performance—introducing associated power, memory sharing, and area penalties—the E8 offers single-core performance of up to 128 TOPS, delivering the computational capability required by the most advanced LLM and ADAS implementations.
Innovative Architecture
The Origin E8 neural engine uses Expedera’s unique packet-based architecture, which is far more efficient than common layer-based architectures. The architecture enables parallel execution across multiple layers achieving better resource utilization and deterministic performance. It also eliminates the need for hardware-specific optimizations, allowing customers to run their trained neural networks unchanged without reducing model accuracy. This innovative approach greatly increases performance while lowering power, area, and latency.
Choose the Features You Need
Customization brings many advantages, including increased performance, lower latency, reduced power consumption, and eliminating dark silicon waste. Expedera works with customers to understand their use case(s), PPA goals, and deployment needs during their design stage. Using this information, we configure Origin IP to create a customized solution that perfectly fits the application.
Market-Leading 18 TOPS/W
Sustained power efficiency is key to successful AI deployments. Continually cited as one of the most power-efficient architectures in the market, Origin NPU IP achieves a market-leading, sustained 18 TOPS/W.
Efficient Resource Utilization
Origin IP scales from GOPS to 128 TOPS in a single core. The architecture eliminates the memory sharing, security, and area penalty issues faced by lower-performing, tiled AI accelerator engines. Origin NPUs achieve sustained utilization averaging 80%—compared to the 20-40% industry norm—avoiding dark silicon waste.
Full TVM-Based Software Stack
Origin uses a TVM-based full software stack. TVM is widely trusted and used by OEMs worldwide. This easy-to-use software allows the importing of trained networks and provides various quantization options, automatic completion, compilation, estimator and profiling tools. It also supports multi-job APIs.
Successfully Deployed in 10M Devices
Quality is key to any successful product. Origin IP has successfully deployed in over 10 million consumer devices, with designs in multiple leading-edge nodes.
Use Case
Custom Network Automotive Deployments
An electric vehicle OEM aimed to develop its own ADAS processor optimized to run both standard and internally developed neural networks and with the flexibility to deploy new networks post-silicon. After testing more than ten different platforms from multiple vendors, the OEM rated Expedera’s ASIL-B readiness-certified Origin IP as the “best-in-market.” This was based on tests that included input resolutions up to 8K and compute capacity ranging from 100 to 500 TOPS, focusing on the lowest deterministic latency and DDR memory bandwidth.
|
|
|
|
|
|
|
|
Compute Capacity | 16K to 64K INT8 MACs |
Multi-tasking | Run >10 Simultaneous Jobs |
Power Efficiency | 18 TOPS/W effective; no pruning, sparsity or compression required (though supported) |
Example Networks Supported | Llama2-7B, YOLO v3, YOLO V5, RetinaNet, Panoptix Deeplab, PlainLite, ResNext, ResNet 50, Inception V3, RNN-T, MobileNet V1, MobileNet SSD, BERT, EfficientNet, FSR CNN, CPN, CenterNet, Unet, ShuffleNet2, Swin, SSD-ResNet34, DETR, others |
Example Performance | YOLO v3 (608 x 608): 626 IPS, 115.6 IPS/W (N7 process, 1GHz, no sparsity/pruning/compression applied) |
Layer Support | Standard NN functions, including Conv, Deconv, FC, Activations, Reshape, Concat, Elementwise, Pooling, Softmax, others. Programmable general FP function, including Sigmoid, Tanh, Sine, Cosine, Exp, others, custom operators supported. |
Data types | INT4/INT8/INT10/INT12/INT16 Activations/Weights FP16/BFloat16 Activations/Weights |
Quantization | Channel-wise Quantization (TFLite Specification) Software toolchain supports Expedera, customer-supplied, or third-party quantization |
Latency | Deterministic performance guarantees, no back pressure |
Frameworks | TensorFlow, TFlite, ONNX, others supported |